How to overcome biases in Artificial Intelligence?
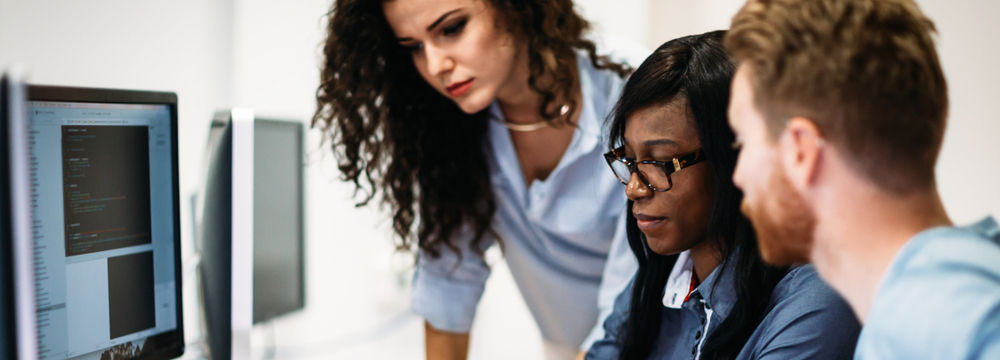
In previous posts, I considered how to overcome biases that hamper diversity, whether as an individual or as an organization. In this post, I no less invite you to the next level: where biases meet Artificial Intelligence. Predictive policing and autonomous weapons systems are a concerning matter. But let’s be honest: in 750 words and within the limits of my authority in AI, we will narrow the discussion on how AI sheds a new light in the workplace. In other words: to which extent AI reinforces existing biases? How improving the interaction between human and machine could benefit to diversity?
Biases in AI and human decision making
In October 2018, Reuter reported that Amazon was scraping its experimental hiring system after uncovering that the ranking of candidates for software developer jobs and other technical posts was gender-biased. This article fueled the controversy about biases in AI. Meanwhile, as researchers from the Institut AI Now of New York University pointed in their April 2019 report: discrimination in AI is not limited to gender. AI systems are also skewed about age, cultural background, sexual orientation, education, and physical disabilities.
Furthermore, the mentioned report highlights the intricacy between biases of data sets used to train the machines and the lack of diversity in the AI industry and academia. Growing evidence shows that the effort to diversify the pipeline neglected workplace cultures and, consequently, failed to increase cognitive diversity. Does that mean that unaddressed human biases definitively pave the way for discrimination in AI?
When AI is an opportunity
Kleinberg et al. advocate that AI might be a source of important gains for disadvantaged groups.
If appropriate regulation can protect against malfeasance in their deployment, then algorithms can become a potentially powerful force for good: they can dramatically reduce discrimination of multiple kinds.
Kleinberg et al.
Those researchers do not deny that biases of those who design the algorithms are a source of concern, but they also bring deeper considerations and offer a framework:
- Thorough (and challenging) screening of the data used to determine when legal remedies should compensate a disparity. In other words, when and how to intervene if a protected group is underrepresented in the distribution.
- Allowing scrutiny thanks to a high degree of transparency: records should be stored and made available for further research
- Adapting to AI specificities the existing legal framework that currently apply to human discrimination
- Making more explicit, articulated (and potentially uncomfortable) choices as algorithms quantify: what is the “weight” of such or such structural disadvantage faced by a particular social group?
- Setting regulations to protect against wrongdoing. The researchers argue that “if appropriate regulation can protect against malfeasance in their deployment, then algorithms can become a potentially powerful force for good: they can dramatically reduce discrimination of multiple kinds.”
This framework has the merit to pose a significant challenge. Pressed by competition and demand of clients for automatized hiring systems, will the stakeholders, by themselves, question the data sets, embrace transparency, enhance multidisciplinary reflection, not to mention, diversify their field? Doubt is permitted.
Awareness and accountability
Facing the potential of AI, the ostrich’s politics would be the worse answer. Whether as a worker, manager, leader, influencer, or enlighted citizen, our ethical compass is at stake.
Information: AI is a headline-grabbing subject. Overcome superficial articles, pore over the subject and develop some personal ideas about fairness, (human) biases, categories, machine-learning, attribution, distribution… Warning: At first, it might be demanding if, like me, you are not a specialist. Some comfort: After a few hours of readings and video-watching, it is totally brain-grabbing. Some organizations provide academic insights: use it.
Insights and resources about AI
> Kate Crawford’s site
and the video You and AI – Machine learning, bias, and implications for inequality
> AI Now Institute from New York University
and the report Discriminating Systems: Gender, Race, and Power in AI
> Partnership on AI
> Turing Institute’s Fairness
Accountability: Fairtrade certifications are more and more visible. Why would fair-AI certifications not be in the interest of all to see the “force of good” foreseen by Kleinberg et al.? If there is a demand for fairness in AI, the market will respond accordingly. Oh dear, this sounds a bit Adam-Smith-biased! As RGPD regulations show, law-makers will have their say too. Meanwhile, for these demand of fairness in AI to arise, human biases must be tackled, and, here, AI can help!
When neurosciences and genetics emerged, some have been tempted to revisit predestination and deny us any free-will. In fact, the more neuronal we are, the more influence have our education, experiences, and interactions with others. The same principle can be applied to AI: the more data and algorithms, the more ethics are implied. The more science, the more human.